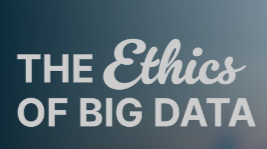
Big Data has revolutionized how organizations collect, analyze, and utilize vast amounts of information to gain insights and make informed decisions. In today’s digital age, where data fuels innovation and drives competitive advantage, ethical considerations surrounding its use have become increasingly critical. This article explores the ethical challenges inherent in Big Data practices, examining privacy concerns. Data security issues, biases in algorithms, and the need for transparency and accountability.
Ethical Issues in Big Data
In the realm of Big Data, several ethical concerns arise due to the sheer volume, velocity, and variety of data being collected and analyzed. These issues encompass privacy, data security, biases in algorithms, transparency, accountability, consent, and data ownership. Addressing these ethical challenges is crucial to harnessing the power of Big Data responsibly.
Privacy Concerns
Privacy is a fundamental ethical issue in Big Data analytics. It pertains to the protection of individuals’ personal information collected through various digital channels. As organizations gather massive datasets from sources such as social media, IoT devices, and transaction records. Concerns about how this data is used and shared without compromising individuals’ privacy rights become paramount. Instances of data breaches and unauthorized access highlight the need for robust privacy frameworks and stringent data protection measures.
Data Security
Alongside privacy, data security remains a critical ethical issue in Big Data. The vast repositories of sensitive information stored by organizations are attractive targets for malicious actors seeking to exploit vulnerabilities for financial gain or to cause harm. Ensuring robust cybersecurity measures, such as encryption, access controls, and regular security audits. This is essential to mitigate the risks of data breaches and uphold user trust.
Bias and Fairness
In Big Data analytics, biases can inadvertently influence decision-making processes, leading to unfair outcomes. Algorithms trained on biased datasets may perpetuate or exacerbate existing social inequalities. For example, biased algorithms in hiring processes might inadvertently discriminate against certain demographic groups. Addressing bias requires careful scrutiny of data collection methods, algorithmic design, and ongoing monitoring to ensure fairness and equity in outcomes.
Transparency and Accountability
Transparency is crucial for maintaining trust in Big Data practices. Users should be informed about how their data is collected, used, and shared. Organizations must adopt transparent policies and practices that disclose data handling procedures and provide individuals with clear, understandable explanations of how their data contributes to analytics and decision-making. Accountability mechanisms ensure that organizations are held responsible for adhering to ethical standards and mitigating potential harms associated with Big Data use.
Consent and Control
Obtaining informed consent from individuals for data collection and usage is a cornerstone of ethical Big Data practices. However, complexities arise when users are unaware of the extent to which their data is collected and how it will be utilized. Empowering individuals with control over their personal data through consent mechanisms, such as opt-in/opt-out choices and data access requests, enhances transparency and respects individuals’ autonomy in deciding how their information is utilized.
Data Ownership
Determining ownership rights over Big Data is another ethical dilemma. While individuals may provide data, organizations often aggregate and derive insights from these datasets. Clarifying ownership rights involves legal and ethical considerations, ensuring that individuals retain control over their personal information while. Allowing organizations to derive value from aggregated data for beneficial purposes, such as improving services or developing new innovations.
Regulatory Frameworks and Guidelines
Regulations like the General Data Protection Regulation (GDPR) in Europe and the California Consumer Privacy Act (CCPA) in the United States set standards for data collection, processing, and storage. However, navigating these regulations can be complex for organizations operating across different jurisdictions. Compliance requires ongoing adaptation to evolving legal requirements and proactive measures to uphold ethical standards in data handling practices.
Case Studies
Examining real-world case studies sheds light on ethical dilemmas faced by organizations leveraging Big Data. For instance, controversies surrounding data breaches at major tech firms underscore the importance of robust cybersecurity measures and accountability in protecting user data. Similarly, ethical concerns arise in healthcare when using patient data for research or personalized medicine. Balancing benefits with privacy protections and consent requirements.
Ethical Decision-Making Framework
Developing an ethical decision-making framework is essential for guiding organizations in navigating complex ethical dilemmas associated with Big Data. Such frameworks emphasize transparency, accountability, fairness, and respect for individuals’ rights. By involving stakeholders, including data subjects, in decision-making processes, organizations can foster trust and uphold ethical principles in data-driven initiatives.
Future Trends in Ethical Big Data Practices
As technology advances, new trends and challenges in ethical Big Data practices emerge. Innovations in artificial intelligence (AI) and machine learning introduce complexities such as algorithmic biases and autonomous decision-making. Ethical considerations will increasingly focus on enhancing algorithmic transparency. Addressing bias through algorithmic auditing, and ensuring that AI systems prioritize fairness and inclusivity.
Conclusion
Ethical considerations lie at the heart of responsible Big Data practices. Balancing innovation with ethical principles ensures that data-driven technologies ethical considerations in big data. Contribute positively to society while respecting individuals’ rights to privacy, fairness, and autonomy. As organizations navigate the complexities of Big Data analytics, they must prioritize transparency. Accountability, and the ethical use of data to build trust with users and stakeholders. By embracing ethical frameworks and regulatory guidelines, businesses can mitigate risks. Foster innovation, and uphold societal values in the digital age.
FAQs About Ethical Considerations in Big Data
- What are the main ethical issues in Big Data? Ethical issues in Big Data include concerns about privacy, data security, biases in algorithms, transparency, consent, and data ownership. These issues arise from the vast amounts of data collected and analyzed, impacting how organizations handle and use personal information.
- How can organizations ensure ethical use of Big Data? Organizations can ensure ethical use of Big Data by implementing transparent data practices. Obtaining informed consent from individuals, prioritizing data security measures, and regularly auditing algorithms for biases. Additionally, complying with relevant regulations and fostering a culture of ethical responsibility among employees are crucial steps.
- What is the role of regulations like GDPR in addressing Big Data ethics? Regulations like the General Data Protection Regulation (GDPR) set standards for how organizations collect, process, and store personal data. GDPR emphasizes principles such as data minimization, purpose limitation, and individuals’ rights to access and control their data. It plays a significant role in protecting individuals’ privacy and promoting ethical practices in Big Data analytics.
- How does bias affect decision-making in Big Data analytics? Bias in Big Data analytics can lead to unfair outcomes by favoring certain groups or perspectives over others. Biased algorithms may perpetuate social inequalities or make inaccurate predictions based on flawed data assumptions. Addressing bias requires careful scrutiny of data sources, algorithm design. Continuous monitoring to ensure fairness and equity in decision-making processes.
Read More :
Business Intelligence