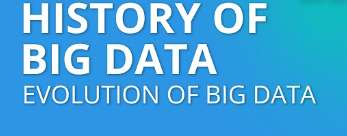
Welcome to the dynamic world of Big Data systems! In an era where information flows faster than ever before, Big Data has emerged as the cornerstone of modern data management and analytics. From revolutionizing industries to shaping how businesses operate, the evolution of Big Data systems has been nothing short of transformative.
Technological Advancements
In the ever-evolving landscape of Big Data, technological advancements have played a pivotal role in shaping how data is stored, processed, and utilized. Let’s explore the key milestones that have propelled Big Data systems to new heights.
Growth of Data Storage Solutions
One of the most significant advancements in Big Data has been the exponential growth in data storage capabilities. From traditional relational databases to modern distributed file systems like Hadoop and cloud-based solutions such as Amazon S3 and Google Cloud Storage, the capacity to store vast amounts of data has expanded exponentially. This growth has been driven by innovations in hardware technology, including the development of high-capacity disk drives and solid-state drives (SSDs), as well as improvements in data compression algorithms that optimize storage efficiency.
Development of Data Processing Techniques
Alongside advancements in storage, the evolution of data processing techniques has revolutionized how organizations extract actionable insights from Big Data. Traditional batch processing methods have given way to real-time data processing frameworks like Apache Spark and Apache Flink, which enable near-instantaneous analysis of streaming data. These frameworks leverage distributed computing paradigms to parallelize data processing tasks across clusters of interconnected machines, significantly reducing processing times and enhancing scalability.
The integration of data processing frameworks with cloud computing platforms has further democratized access to scalable computing resources, allowing organizations of all sizes to harness the power of Big Data without heavy upfront investments in infrastructure. Cloud-based data processing services like Amazon EMR and Google Dataflow provide managed environments for running distributed data processing jobs, simplifying deployment and management for data engineers and analysts alike.
Challenges and Solutions
While Big Data systems offer unprecedented opportunities for data-driven decision-making, they also present unique challenges that organizations must navigate to maximize their potential. Let’s delve into some of the key challenges and the innovative solutions that address them.
Data Privacy and Security Issues
One of the foremost concerns surrounding Big Data is the protection of sensitive information against unauthorized access and breaches. As organizations collect and analyze vast amounts of data from diverse sources, ensuring data privacy becomes increasingly complex. Regulatory frameworks like GDPR and CCPA have imposed stringent requirements for data protection, compelling businesses to implement robust security measures and adhere to strict compliance guidelines.
To mitigate these challenges, organizations are adopting a multi-layered approach to data security. This includes encryption techniques to safeguard data both at rest and in transit, access control mechanisms that limit data access based on user roles and permissions, and anonymization methods to protect individual identities while preserving data utility for analysis. Additionally, continuous monitoring and auditing of data access patterns help detect and respond to potential security threats in real time, ensuring proactive protection against cyber threats.
Collaborative efforts between industry stakeholders, government bodies, and cybersecurity experts are also instrumental in addressing emerging data privacy challenges. By fostering a culture of data stewardship and transparency, organizations can build trust with consumers and stakeholders, reinforcing their commitment to ethical data practices.
Scalability and Infrastructure Constraints
Another significant challenge in Big Data systems is the ability to scale infrastructure to accommodate growing data volumes and processing demands. Traditional on-premises data architectures often struggle to keep pace with the exponential growth of data, leading to performance bottlenecks and operational inefficiencies.
Cloud computing has emerged as a game-changer in addressing scalability challenges by providing elastic and scalable infrastructure on-demand. Platforms like Amazon Web Services (AWS), Microsoft Azure, and Google Cloud Platform offer scalable storage and computing resources that can be dynamically provisioned based on workload requirements. This flexibility allows organizations to scale their evolution of big data systems operations seamlessly. From pilot projects to enterprise-wide deployments, without upfront capital investments in hardware.
Future Trends
The future of Big Data systems holds exciting prospects as technology continues to evolve and innovate. Let’s explore some of the emerging trends that are shaping the landscape of data analytics and decision-making.
AI-driven Insights
Artificial Intelligence (AI) is set to revolutionize how organizations extract insights from Big Data. AI-powered analytics algorithms can autonomously analyze vast datasets, uncovering hidden patterns, correlations, and trends that human analysts may overlook. Machine learning models, trained on historical data, enable predictive analytics and prescriptive recommendations. Empowering businesses to make data-driven decisions with unprecedented accuracy and foresight.
Edge Computing and Real-time Analytics
The proliferation of Internet of Things (IoT) devices and sensors is driving demand for real-time data processing at the network edge. Edge computing platforms bring computational resources closer to data sources, minimizing latency and enhancing responsiveness for time-sensitive applications. This paradigm shift allows organizations to analyze streaming data streams in real-time. Enabling immediate decision-making and actionable insights at the point of data generation.
Edge computing also reduces reliance on centralized data centers and cloud infrastructure, improving scalability and resilience in distributed environments. By leveraging edge analytics, organizations can address bandwidth constraints, comply with data sovereignty regulations. Enhance user experiences evolution of big data systems in remote or resource-constrained locations.
Conclusion
As we wrap up our exploration of the evolution of Big Data systems, one thing is clear. The journey has been nothing short of remarkable. From humble beginnings to becoming a cornerstone of modern data-driven decision-making. Big Data has reshaped industries, fueled innovation, and empowered organizations to navigate an increasingly complex digital landscape.
FAQs about Big Data Systems
What are the main challenges in implementing Big Data systems?
Implementing Big Data systems can be complex due to several challenges. One major challenge is handling the sheer volume, velocity, and variety of data generated from various sources. This requires robust infrastructure and scalable solutions to store, process, and analyze data efficiently. Another challenge is ensuring data privacy and security, especially with stringent regulations like GDPR and CCPA in place. Organizations must implement strong encryption, access controls, and data anonymization techniques to protect sensitive information.
How has Big Data evolved over the years?
Big Data has evolved significantly from its early days of relational databases and batch processing to today’s era of real-time analytics, AI-driven insights, and edge computing. Technological advancements have expanded data storage capacities, improved processing speeds, and enabled sophisticated analytics capabilities. The integration of machine learning and AI has revolutionized how organizations derive insights from data. Making predictive analytics and personalized recommendations a reality.
What are some industries benefiting most from Big Data?
Several industries are leveraging Big Data to gain competitive advantages and drive innovation. Healthcare is using Big Data for patient diagnostics, personalized medicine, and epidemiological studies. Finance utilizes Big Data for fraud detection, risk management, and algorithmic trading. Retail and e-commerce companies leverage Big Data for customer segmentation, personalized marketing, and supply chain optimization. Additionally, sectors like manufacturing, telecommunications, and energy are also benefiting from data-driven insights to improve efficiency and decision-making.
What are the future trends in Big Data systems?
The future of Big Data systems is promising with trends like AI-driven insights, real-time analytics at the edge. The proliferation of IoT devices evolution of big data systems. AI will continue to enhance predictive analytics and automate decision-making processes. Edge computing will enable real-time data processing and reduce latency for time-sensitive applications. Advancements in data privacy, ethical AI, and hybrid cloud architectures are expected to shape the future landscape of Big Data systems.
How does Big Data integrate with AI and machine learning?
Big Data and AI are closely intertwined, with Big Data providing the raw material—vast amounts of data—and AI techniques such as machine learning algorithms extracting actionable insights from this data. Machine learning models learn from historical data to make predictions, classify patterns, and optimize processes. Big Data platforms provide the scalability and computational power necessary to train and deploy AI models effectively. Enabling organizations to harness the power of data-driven decision-making and innovation.
Read More :